Solcast’s solar forecast accuracy just got even better in North America.
Our data science team has recently integrated NOAA’s High-Resolution Rapid Refresh (HRRR) model as an input into our cloud, irradiance and power forecasts. This integration is designed to enhance the accuracy of the solar forecasts required to manage your assets amidst weather variability.
This enhancement is now live and accessible via the Solcast API, allowing you to retrieve higher resolution and more accurate solar forecasts, with a relative ~5% error improvement for forecasts from +2h to +48h, for your asset and grid operations. Earlier lead times up to +2h continue to use our industry-leading satellite “nowcast” forecasting system. Our historic forecast archive has also been updated with these new HRRR inputs.
Integrating HRRR in the Solcast API
The rise of rapid-update mesoscale weather models presents an opportunity to enhance the accuracy of forecasts we deliver to our partners and customers in the solar industry. At Solcast, we carefully select from multiple data inputs for our models, testing which models, parameters, and machine-learning algorithms enhance accuracy.

The HRRR model stands out due to its 3 km resolution, a significant improvement over the 25 km resolution of the GFS models. This high resolution, combined with increased update frequency, provides more detailed forecasts. It will become more granular soon, as we are updating our models to take advantage of hourly updates on intra-day forecasts from the HRRR model. These updates offer near real-time insights that are crucial for efficient energy management and decision making.
Improving accuracy by combining HRRR inputs with Solcast modeling
Whilst increased resolution provides a more physically realistic view, it is not always more accurate. Higher resolution models can sometimes depict detailed features that do not correspond to actual weather conditions, resulting in “false precision” and paradoxically higher error than coarser models.
To maximize the benefits of HRRR’s high resolution model, we apply a series of correction and machine-learning algorithms to filter out these inaccuracies. Solcast extracts meaningful weather data from HRRR and combined with our state-of-the-art clear-sky radiation model we train against quality-controlled site measurements and Solcast satellite actuals. This process significantly reduces errors from HRRR raw inputs, which is then combined with other Solcast post-processed models (including GFS and ECMWF weather models) to produce the final Solcast forecast.
To further enhance forecasting accuracy, we have synchronized Solcast forecast updates with each HRRR forecast issue, which updates more frequently compared to other models.
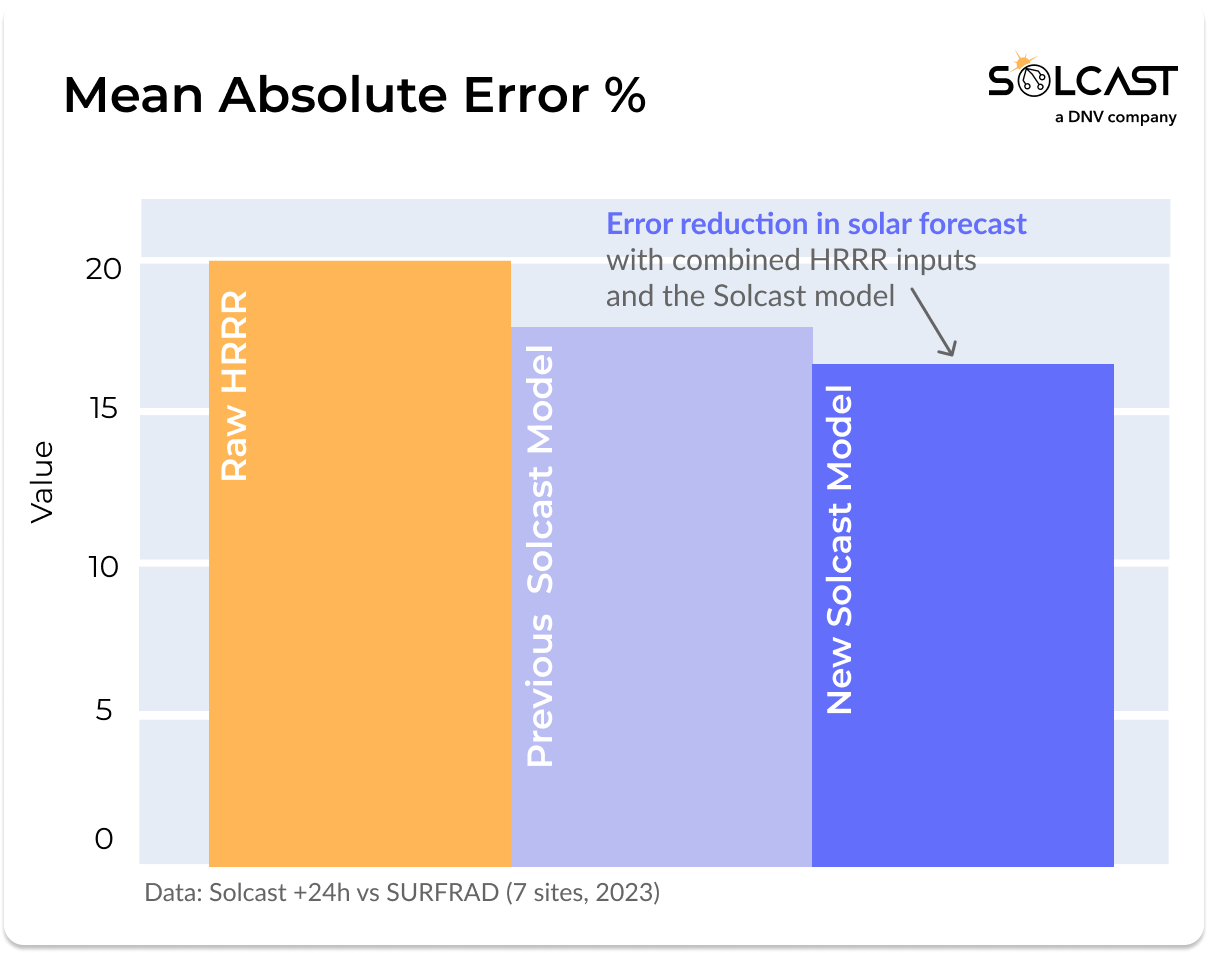
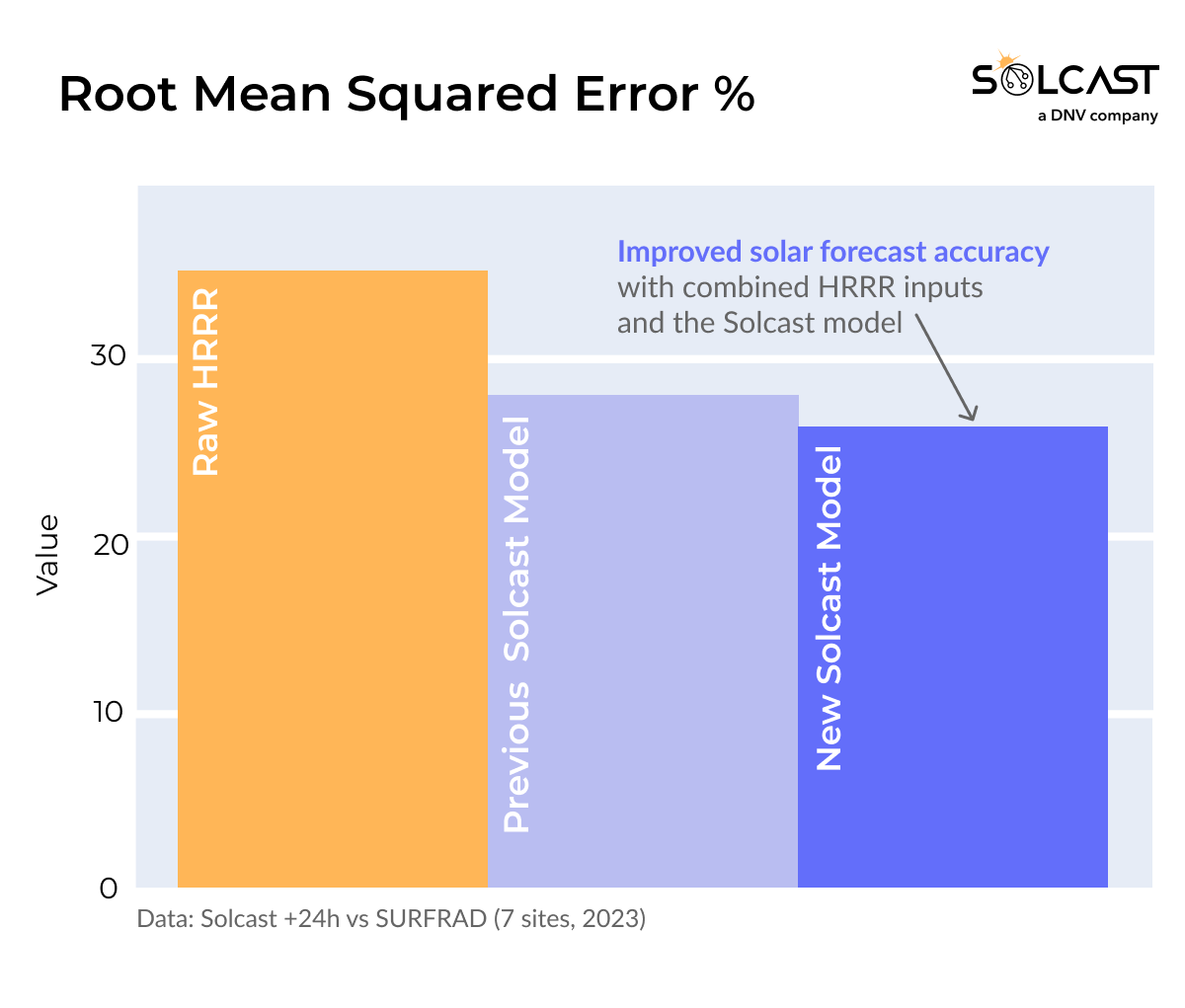
Get More Accurate Solar Forecasts for Your Assets
As the solar industry grows exponentially, precise solar forecasting becomes increasingly critical for efficient asset operations.
Understanding these challenges, we continuously refine our models, including the integration of HRRR and the recently added ICON-EU model in Europe. These enhancements towards data accuracy help you make timely, informed decisions to optimize energy output, manage risk management, and enhance operational efficiency.
See the difference in our data accuracy when you sign up for a Solcast API toolkit. Reach out to our team for a free guided trial.
